Primary Data Collection Methods in Agriculture Research
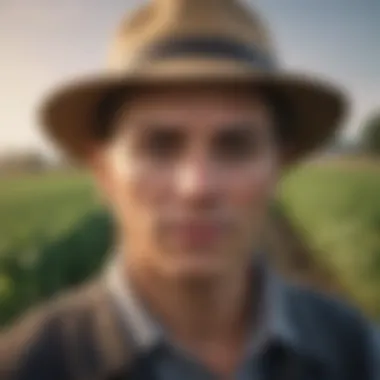
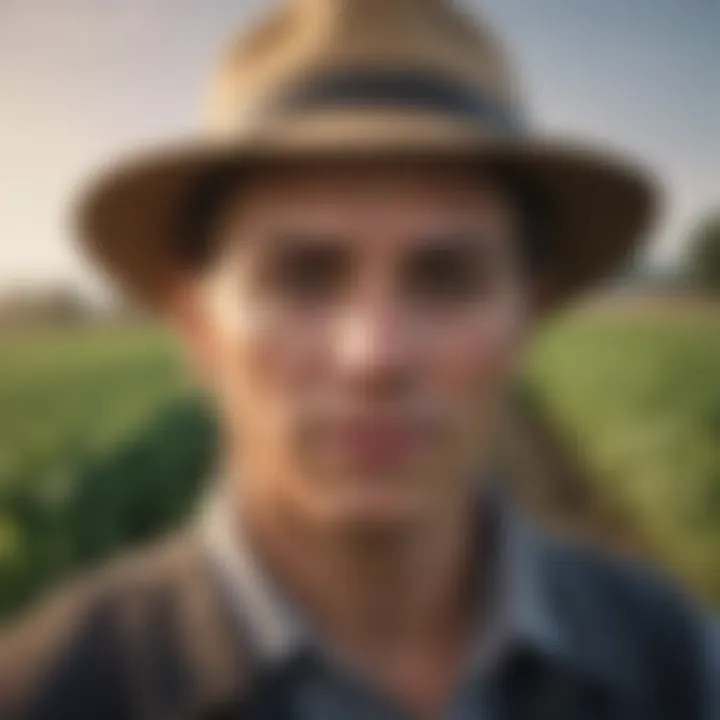
Intro
In the field of agriculture and horticulture research, the collection of primary data is vital. This data informs important agricultural practices and innovations. By understanding different collection methods, researchers, practitioners, and enthusiasts can enhance their approaches. The selection of appropriate methods affects the validity and reliability of the research.
The article will first define essential concepts and terminology connected to data collection. Understanding these terms is imperative for comprehending the methods that follow. Next, current trends and innovations will be examined. Here, a focus will be on technological advancements that aid data collection and promote sustainable practices.
Further, best practices and techniques will be outlined in a step-by-step manner. This section provides practical guidance on how to implement effective data collection methods in agricultural studies. Overall, the article aims to offer a well-rounded perspective, enabling better research practices that contribute to sustainable agriculture.
Key Concepts and Terminology
Definition of Terms
Primary data refers to original data gathered directly from the source for a specific purpose. In agriculture, this could mean information collected through observations on crop yield or farmer interviews. Secondary data, in contrast, refers to data that has been previously collected and processed by others.
In addition to these definitions, qualitative data involves non-numerical information, often obtained through interviews or focus groups. Quantitative data comprises numerical values and can be analyzed statistically, which is crucial in assessing agricultural productivity and effectiveness.
Importance in Agriculture
The significance of primary data collection is profound. Accurate data collection allows for informed decision-making, resource management, and policy development in agricultural practices. As farmers face challenges like climate change and market fluctuations, effective data collection helps in creating responsive strategies.
"Informed decisions in agriculture lead to sustainable practices and better yield outcomes."
The ability to adapt to new information is crucial. Employing diverse data collection methods can yield a comprehensive understanding of farming challenges.
Current Trends and Innovations
Technological Advancements
Emerging technologies are transforming data collection processes. Precision agriculture utilizes various tools like drones, sensors, and GPS. These technologies enhance the ability to gather and analyze data efficiently.
For instance, the adoption of soil moisture sensors allows farmers to obtain real-time data about soil conditions, thus optimizing irrigation practices. Similarly, mobile applications can facilitate data entry and analysis on the go, making research more accessible.
Sustainable Practices
Sustainable practices in agriculture benefit from robust data collection. By analyzing data on crop rotations, pest management, and resource utilization, agriculturalists can make informed choices that promote ecological balance. Such practices ensure long-term productivity while preserving environmental health.
Best Practices and Techniques
Step-by-Step Guides
To effectively collect primary data in agriculture and horticulture, following structured steps is essential:
- Define Research Objectives: Clearly outline what you aim to achieve with your study.
- Select Appropriate Methods: Choose between surveys, interviews, or experiments based on your objectives.
- Train Data Collectors: Ensure that individuals collecting data understand the process thoroughly.
- Pilot Test Your Methods: Conduct a small-scale trial to identify potential issues before full implementation.
- Analyze Collected Data: Use statistical tools to interpret the data and draw conclusions.
Tools and Resources
Several tools can aid in data collection:
- Qualtrics and SurveyMonkey: For conducting online surveys.
- Google Forms: A free tool for creating surveys quickly.
- Tableau: Useful for data visualization once data is collected.
For more information on agricultural practices and technologies, visit Wikipedia or Britannica.
Prelude to Primary Data Collection
Primary data collection is a fundamental component of agriculture and horticulture research. Gathering first-hand information is crucial for addressing specific research questions and understanding the complexities of farming practices. This process enables researchers to build their findings on solid foundations, ensuring that the data is relevant and applicable to real-world scenarios.
The significance of effective primary data collection can be seen in its various forms, such as surveys, interviews, or observational studies. Each method offers unique advantages and should be carefully chosen based on the objectives of the research. Collecting primary data helps to ensure that research findings are valid and reliable, allowing for better decision-making.
Effective data collection can lead to insights that drive sustainable agricultural practices. This enhances productivity while reducing environmental impacts, aligning with the current demands of global food production. By understanding the different data collection methods, farmers and researchers can tackle challenges in agriculture more effectively, paving the way for innovations and improvements in the field.
Definition of Primary Data
Primary data refers to original information collected for a specific research purpose. Unlike secondary data, which consists of previously collected information from other sources, primary data originates from direct experiences or observations. In agriculture and horticulture research, this often involves gathering data through firsthand methods such as surveys or interviews conducted with participants directly involved in farming.
Researchers often choose primary data because it is typically more accurate and relevant to their needs. It allows for the incorporation of the latest trends, techniques, and opinions from those engaged in agriculture at various levels, from small farmers to large agricultural corporations.
Importance in Research
The importance of primary data in research cannot be overstated. It provides the basis for understanding context, motivations, and challenges faced by farmers. Collecting primary data helps researchers to:
- Identify and analyze specific issues related to agriculture and horticulture.
- Develop tailored recommendations that address the needs of farmers.
- Examine variations across different geographical areas or farming practices.
- Ensure the reliability of findings by relying on newly collected, relevant information.
Moreover, utilizing primary data collection methods promotes engagement with the agricultural community. It allows researchers to hear diverse perspectives and incorporate them into their findings. This emphasis on direct input can foster a better understanding of the agricultural landscape, ultimately impacting practices in positive and sustainable ways.
Qualitative vs. Quantitative Data
In agriculture and horticulture research, the distinction between qualitative and quantitative data is pivotal. Each type offers unique advantages and suits different research objectives. Understanding these differences allows researchers to tailor their methods effectively, ensuring robust findings that can influence agricultural practices.
Characteristics of Qualitative Data
Qualitative data provides in-depth insights into perceptions, experiences, and behaviors. It is primarily descriptive, focusing on qualities rather than quantities. Here are key characteristics:
- Descriptive Nature: Qualitative data is often gathered through methods like interviews and focus groups, capturing the nuances of human experiences.
- Contextual Understanding: This type of data helps researchers explore the context behind agricultural practices. For instance, understanding farmer motivations or beliefs about sustainability.
- Flexibility: The process of gathering qualitative data can adapt as the research unfolds, allowing for emergent themes to be explored.
- Subjectivity: It often involves interpretation, making findings more subjective compared to quantitative methods.
Given these characteristics, qualitative insights can lead to deeper understanding and inform policy decisions in agriculture and horticulture.
Characteristics of Quantitative Data
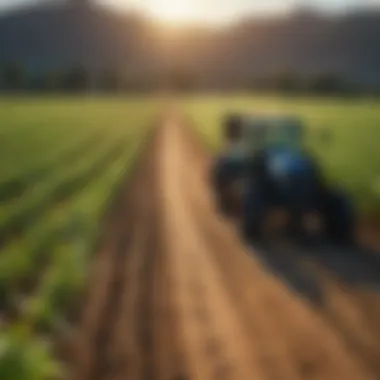
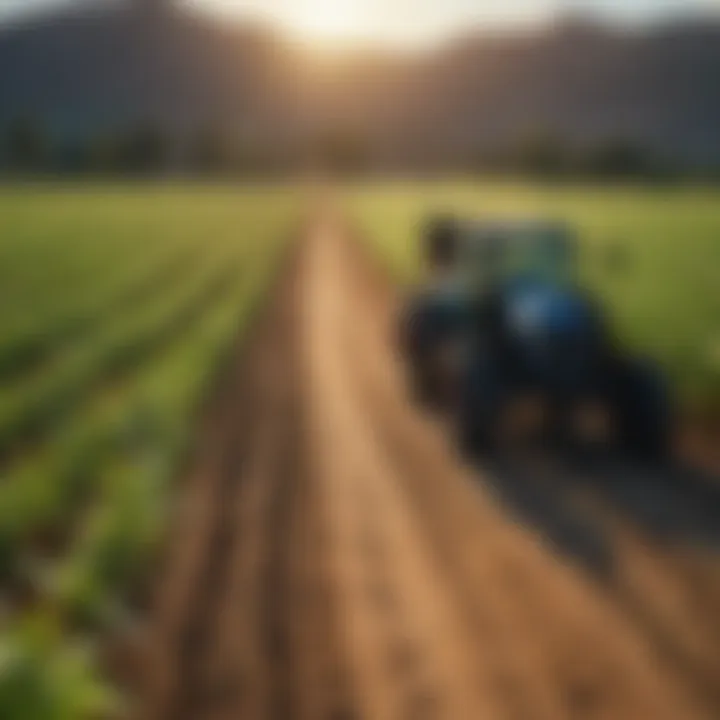
Quantitative data focuses on numerical metrics, allowing for statistical analysis. This data type is essential when researchers need measurable evidence to support claims. Key characteristics include:
- Objective Measurement: Quantitative data relies on figures, making it more straightforward to analyze. This includes yields, growth rates, and environmental measurements.
- Statistical Analysis: Researchers can apply various statistical techniques for hypothesis testing, thus validating findings with data.
- Generalizability: The findings can often be expanded to a larger population, especially when using random sampling techniques.
- Structured Approach: Data collection is typically more controlled, utilizing surveys and experiments to minimize bias.
In summary, both qualitative and quantitative data play significant roles in agricultural research. While qualitative data enhances understanding of complex phenomena, quantitative data provides measurable evidence necessary for scientific validation. Balancing both types can lead to comprehensive research outcomes that drive effective agricultural practices.
Surveys and Questionnaires
Surveys and questionnaires are critical tools in gathering primary data in agriculture and horticulture research. They allow researchers to collect information from a large number of participants efficiently. This method can uncover insights that are not immediately visible through observational methods. Furthermore, the design and implementation of surveys can significantly influence the quality of data obtained, making it essential to understand the nuances involved.
The flexibility of surveys is notable. They can be tailored to address specific research questions while also allowing for broad data collection across different demographic groups. This adaptability makes them vital for understanding the diverse factors affecting agriculture and horticulture practices.
Designing Effective Surveys
To design an effective survey, clarity and conciseness are key. Researchers must ensure that questions are straightforward and relevant to the study objectives. Good survey design also involves testing the survey on a small sample before wider distribution to identify any confusing elements or biases.
Effective surveys should have a logical flow, guiding respondents through questions in a coherent manner. This organization prevents confusion and encourages participants to provide thoughtful responses. By clearly defining the objectives and the target audience, researchers can create surveys that yield actionable insights.
Types of Questions
Closed-Ended Questions
Closed-ended questions are a common feature in surveys, allowing for straightforward answers, typically in the form of yes/no, multiple-choice, or scale ratings. These questions enable researchers to quantify responses and simplify data analysis. They are beneficial because they maximize the likelihood of getting a response and reduce the complexity of analysis.
One key characteristic of closed-ended questions is their ability to facilitate statistical analysis. This is particularly valuable in agricultural research, where trends and patterns across large data sets can inform best practices or intervention strategies. The challenge with closed-ended questions, however, lies in their potential to miss nuances in respondents’ opinions and experiences.
Open-Ended Questions
Open-ended questions invite respondents to share their thoughts freely, providing richer qualitative data. This method is particularly useful in uncovering unexpected insights or diverse opinions on agricultural practices. The key characteristic of open-ended questions is the depth of information they can yield. They encourage detailed responses that can highlight issues not covered by closed-ended options.
However, the analysis of open-ended responses can be time-consuming and complex. Researchers must categorize and interpret a wide range of answers, which can introduce bias if not done carefully. While open-ended questions provide valuable context, they require careful thought in survey design to avoid overwhelming respondents and to ensure clarity in responses.
Sampling Techniques
Random Sampling
Random sampling is a technique where every member of a population has an equal chance of being selected. This method enhances the representativeness of the sample, which is vital for valid conclusions in research. A key characteristic of random sampling is that it minimizes selection bias and ensures that results can be generalized to a larger population.
This technique is popular among researchers in agriculture because it can yield a sample that accurately represents the diverse farming community. However, random sampling requires careful planning and execution to ensure that it is truly random and that the sample size is sufficient for reliable results.
Stratified Sampling
Stratified sampling involves dividing the population into subgroups, or strata, before selecting samples from each group. This method ensures that specific segments are adequately represented in the sample, which is crucial when different groups within a population may exhibit varied behaviors or responses.
A key characteristic of stratified sampling is its ability to provide more precise estimates by capturing the diversity within a population. This makes it a beneficial choice when researching diverse agricultural practices that vary by region or socioeconomic status. However, stratified sampling can be more complex to implement than random sampling, requiring detailed knowledge of the population to define the strata effectively.
Interviews
Interviews are a vital primary data collection method in agriculture and horticulture research. They provide valuable insights directly from individuals with firsthand experience or knowledge. Interviews can capture the complexities of human thought and perception, which quantitative methods might miss. By utilizing interviews, researchers can delve into the subjective experiences, opinions, and motivations of participants, leading to a more nuanced understanding of the agricultural landscape.
There are specific elements that enhance the effectiveness of interviews in research. Firstly, they allow for in-depth discussions, enabling researchers to follow up on responses. This adaptability can lead to richer data, as unexpected topics may arise. Furthermore, interviews can build rapport with respondents, leading to more honest and thoughtful answers. This level of engagement can uncover valuable information that informs better agricultural practices and innovations.
Structured Interviews
Structured interviews are characterized by a fixed set of questions that are asked in a specific order. This method provides consistency across interviews, making it easier to compare responses. They are particularly useful when the researcher seeks to gather specific information or if the sample size is large. Structured interviews reduce variability, which is essential for quantitative analysis.
The benefits of structured interviews include the ease of data processing and higher reliability. Data can be easily codified and quantified, which allows for straightforward statistical analysis. However, this method may limit the depth of responses. Participants may feel constrained by the rigid framework, leading them to withhold insights or elaborate less on their opinions. Thus, while structured interviews yield reliable data, they may miss out on rich, qualitative insights.
Semi-Structured Interviews
Semi-structured interviews combine both fixed questions and the flexibility to explore topics as they arise. In this approach, the interviewer prepares a set of guiding questions while remaining open to advancing the conversation based on respondents’ answers. This method is particularly beneficial in agriculture and horticulture research as it allows researchers to gain in-depth insights while still adhering to a general structure.
Semi-structured interviews facilitate a balance between reliability and depth. They enable the researcher to remain focused on specific topics while allowing room for unexpected insights. This adaptability can lead to discoveries that structured interviews might not uncover. However, it requires more skill and experience from the interviewer to navigate the discussion effectively without losing the thread of the research objectives.
Unstructured Interviews
Unstructured interviews are the least formal type of interviews. In this approach, the conversation flows organically, with the interviewer guiding without a fixed set of questions. This method is useful for exploratory research, where understanding the broader context or discovering new themes is the goal.
The primary benefit of unstructured interviews is their ability to generate rich, qualitative data. Participants have the freedom to express their thoughts comprehensively, leading to potentially unexpected findings. However, they can also pose challenges. There is a risk of inconsistency, making data analysis complex and less reliable. Researchers must sift through vast amounts of qualitative data, which can be time-consuming.
Observational Methods
Observational methods hold significant value in agriculture and horticulture research. They allow researchers to gather data in real-time, leading to insights that might not surface through other data collection techniques. Observational methods are essential in capturing the dynamics of agricultural practices, crop forms, pest behaviors, and overall ecosystem interactions. These methods contribute to a clearer understanding of how specific agricultural techniques affect yield, quality, and sustainability.
Researchers can utilize observational methods to get contextually rich data. The two primary types of observational research are participant observation and non-participant observation. Each serves distinct purposes, adapting to different research needs and contexts. When implementing these methods, researchers must pay careful attention to ethical considerations, environmental conditions, and potential biases that might influence their findings.
Participant Observation
Participant observation is a technique where the researcher immerses themself in the environment being studied. In agricultural settings, this may involve spending time on farms, working alongside farmers, and engaging in daily activities. This type of observation enables the researcher to gain in-depth knowledge about farming practices, social interactions, and cultural contexts.
Benefits of participant observation include:
- Rich context: It provides detailed insights into daily operations, customs, and challenges faced by farmers.
- Building trust: Farmers might be more open and honest when interacting with someone actively involved in their work.
- Nuanced data: Researchers can capture subtleties that surveys or interviews may miss.
However, maintaining objectivity can be a challenge. The researcher's presence may alter the behavior of those being observed. Therefore, it is crucial to document personal biases while gathering information.
Non-Participant Observation
Non-participant observation involves observing subjects without direct involvement in their activities. This method enables researchers to study behaviors and interactions in a more natural state. For instance, a non-participant observer might watch how farmers apply irrigation techniques or manage pest control without intervention.
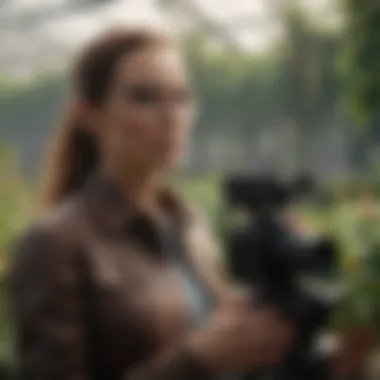
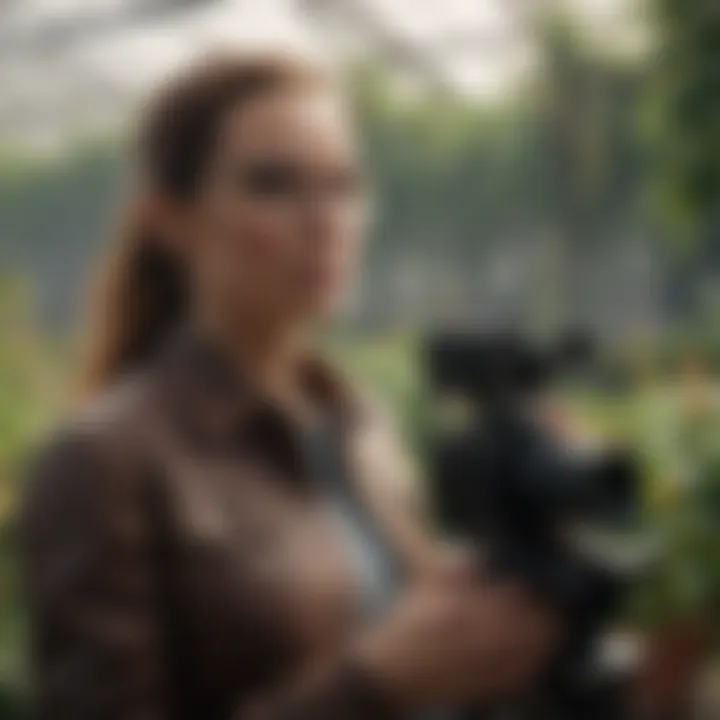
The advantages of non-participant observation include:
- Objective data: The observer can collect data without impacting the subjects, leading to more reliable findings.
- Larger sample size: Non-participant observations can cover multiple locations and gather data on a broader scale.
- Focused research: Researchers can concentrate on specific variables or phenomena without getting involved in the daily operations.
However, this approach can sometimes lack the context provided by participant observation. Researchers should be aware of the limitations regarding the depth of understanding that can be achieved without engagement.
In summary, observational methods are crucial in agriculture and horticulture research. Both participant and non-participant approaches offer unique perspectives that, when combined, can lead to comprehensive insights and knowledge. Incorporating these methods effectively can enhance research quality and relevancy.
Experimental Methods
Experimental methods form a crucial part of primary data collection in agriculture and horticulture research. These methods enable researchers to establish causal relationships between variables, offering insights that can enhance agricultural practices. By systematically manipulating factors and observing outcomes, researchers can effectively assess interventions, treatments, or conditions that impact crop and plant performance. The results derived from experimental methods often hold the power to inform best practices in sustainable farming and contribute to food security.
In the context of agriculture, experimental methods can be divided into two primary categories: field experiments and controlled experiments. Each offers unique advantages that help tailor the research to specific objectives, while also presenting distinct considerations that researchers must navigate.
Field Experiments
Field experiments are conducted in real-world agricultural settings, allowing farmers and researchers to observe the effects of various treatments under natural conditions. These experiments provide valuable data regarding plant growth, yield, and other performance metrics that are relevant to commercial farming. One key advantage of field experiments is their ability to account for environmental variability, which is critical for understanding how different factors influence agricultural outcomes.
However, implementing field experiments comes with its own set of challenges. Factors such as weather, pests, and soil variability can introduce confounding variables that may skew results. To mitigate these issues, it is essential for researchers to adopt stratified sampling techniques and ensure that experiments are replicated across different locations and conditions.
Some important aspects to consider when conducting field experiments include:
- Realism: Provides insights in actual farming conditions.
- Replication: Ensures results are consistent and reliable.
- Time and Resource Intensive: Requires careful planning and resource allocation.
Controlled Experiments
Controlled experiments occur within a controlled environment, such as greenhouses or laboratories. This method allows researchers to isolate specific variables, minimizing the impact of extraneous factors that may otherwise influence the results. Controlled experiments are particularly useful for testing hypotheses related to biological processes or chemical interactions in plants.
The precision of controlled experiments can yield high-quality data that lends itself well to statistical analysis. They often enable researchers to pinpoint the exact nature of the relationships between treatments and outcomes, providing clear recommendations for practitioners. Despite their advantages, controlled experiments can sometimes lack realism, making it difficult to extrapolate findings to real-world agricultural settings.
Key considerations for controlled experiments include:
- Isolation of Variables: Helps identify direct cause-and-effect relationships.
- Limited Contextualization: Findings may not always translate to field conditions.
- High Control Over Environment: Reduces randomness in experiments.
In summary, both field and controlled experiments serve as vital tools in agricultural research. They equip researchers with insights that can guide decision-making and optimize processes in agriculture and horticulture. Ultimately, choosing the appropriate experimental method hinges on the specific research question and the nature of the anticipated results.
Focus Groups
Focus groups serve as a critical tool in primary data collection within agriculture and horticulture research. They provide a platform for gathering in-depth opinions and insights from participants who share a common interest or experience related to agricultural practices or horticultural methods. This qualitative method enables researchers to explore complex topics in a rich context, making it possible to obtain nuanced information that other quantitative methods may overlook.
The importance of focus groups lies in their ability to foster dynamic conversations among participants. They can unearth collective perspectives on issues such as crop management, pest control strategies, or sustainable practices. By emphasizing the interactions and discussions within the group, researchers gain a deeper understanding of how various factors influence decision-making processes in agriculture.
Defining Focus Groups
Focus groups can be defined as moderated discussions involving a small group of people, typically ranging from six to twelve participants. Each group is selected based on specific characteristics relevant to the research topic. For instance, participants might be local farmers, agricultural scientists, or even consumers interested in sustainable farming practices. The moderator guides the conversation, directing it towards key topics and ensuring that all voices are heard.
The primary goal of focus groups in agricultural research is to obtain qualitative insights that inform our understanding of farming practices and challenges. Unlike standard surveys where the interaction is limited to individual responses, focus groups allow for a collaborative environment where participants can build on each other's ideas and experiences. This method captures dialogue and reactions, making it easier to identify common themes and varied perspectives within the agricultural community.
Facilitating Discussions
Facilitating focus group discussions involves careful planning and execution. A skilled moderator plays an essential role in establishing a comfortable atmosphere where participants feel encouraged to speak openly. Several factors contribute to effective facilitation:
- Preparation of Discussion Guides: Developing a structured guide with open-ended questions helps steer the conversation while allowing flexibility. This balance is critical for exploring unexpected insights.
- Creating a Safe Environment: Participants must feel safe to express their views without fear of judgment. Building rapport is necessary to encourage active participation.
- Engaging All Participants: The moderator should direct questions to different individuals, ensuring everyone contributes. This prevents dominance by more vocal participants.
- Using Prompts: When discussions stall, prompts can revitalize the conversation, helping to reach deeper levels of discussion.
The outcome of well-facilitated focus groups is valuable qualitative data that can guide future agricultural research, policy decisions, and community practices. With careful facilitation, a focus group can illuminate complex agricultural issues and foster collaborative solutions in the field of agriculture.
Ethical Considerations in Data Collection
Ethical considerations play a crucial role in primary data collection within agriculture and horticulture research. They ensure that the rights and dignity of all participants are respected. As researchers gather data, they navigate a landscape that is not only technical but also moral. Ethical practices can enhance the trustworthiness and credibility of the research. Thus, understanding these considerations can significantly benefit the research outcomes, aligning them with broader societal values.
Informed Consent
Informed consent is a fundamental principle in ethical data collection. This process involves providing potential participants with all necessary information regarding the study. Participants should understand the research's purpose, what data will be collected, and how it will be utilized. Also, they should be made aware of any possible risks associated with their involvement. Only after this clear communication can they agree to participate, ensuring that their consent is truly informed.
Benefits of Informed Consent:
- Builds trust between researchers and participants.
- Facilitates transparency in the research process.
- Respect the autonomy of participants, allowing them to make educated decisions.
- Reduces the likelihood of ethical violations that could undermine research integrity.
Data Privacy
Data privacy is another critical concern. It focuses on safeguarding the personal information collected from participants. In agriculture and horticulture research, participants might share sensitive information about farming practices or personal experience. Researchers must implement strong measures to protect this data from unauthorized access. This may include using data anonymization techniques, secure storage systems, and limiting data access to authorized personnel only.
Key Considerations for Data Privacy:
- Confidentiality: Ensuring that personal information is never disclosed without consent.
- Data Minimization: Collecting only the information necessary for the research.
- Responsibility: Researchers must understand their role in protecting participant data.
"Ethical data collection is not just about compliance; it enhances the quality and reliability of research findings."
These ethical considerations are more than mere procedural formalities. They form the foundation of responsible research practices that empower participants while generating valuable insights for the agricultural community.
Challenges in Primary Data Collection
In agricultural and horticultural research, the process of primary data collection comes with its own set of challenges. Identifying these challenges is crucial for researchers and practitioners. Understanding these can help ensure that studies yield valid and reliable results.
Sampling Bias
Sampling bias occurs when the selected sample does not accurately represent the population being studied. This can happen due to various factors, such as the method of selection or the geographic limitations of the study area. For instance, if a survey targets only large commercial farms, the data collected may not reflect the experiences or needs of smaller farms or home gardeners.
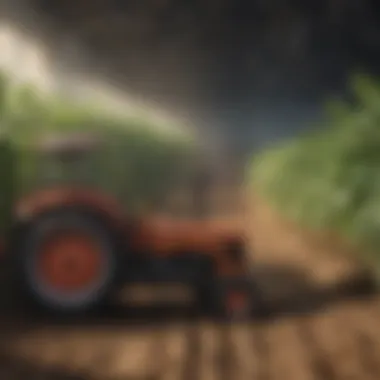
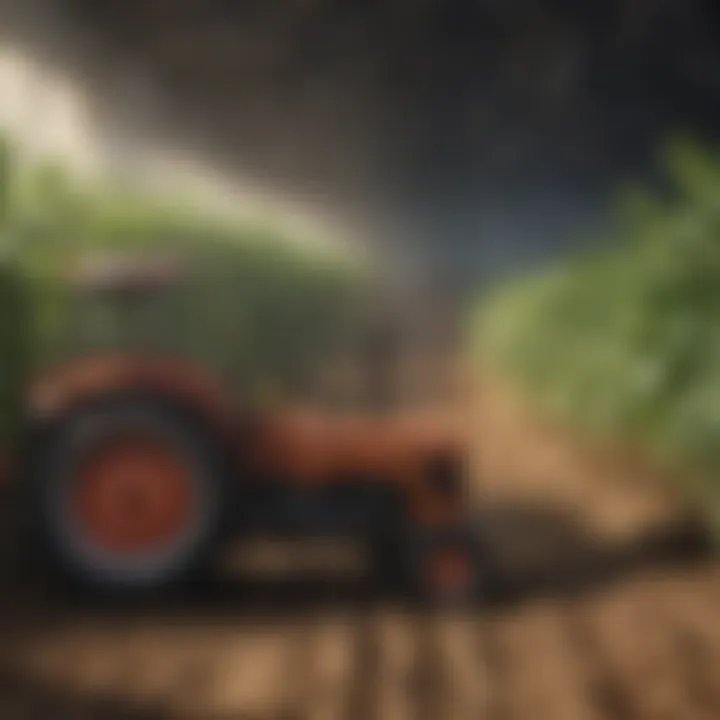
Addressing sampling bias can significantly improve the quality of data collected. Here are some strategies to mitigate this bias:
- Diverse Sampling Techniques: Using techniques like stratified sampling can help ensure various subgroups within the population are represented.
- Adjust Survey Reach: Expanding the geographic area and including different types of farmers might yield a more comprehensive understanding of agricultural practices.
- Regular Review of Methods: Continuously reviewing sampling methods helps adapt to changes in the agricultural landscape, ensuring ongoing relevancy.
By focusing on reducing sampling bias, researchers can enhance the credibility of their data, bridging gaps that may exist in understanding agricultural challenges.
Response Bias
Response bias refers to the tendency of respondents to answer questions untruthfully or misleadingly. This may stem from social desirability, misunderstanding questions, or even a lack of knowledge about the topic. In agriculture, this can be quite problematic, as inaccurate responses may lead to misguided conclusions.
To tackle response bias, researchers should consider these essential tactics:
- Clear and Concise Questions: Simplifying questions allows respondents to understand them better, reducing confusion.
- Anonymity Assurance: Assuring respondents of anonymity can encourage them to answer more honestly, especially on sensitive topics.
- Pilot Testing: Conducting a pilot study can help identify potential biases in questions before the actual data collection.
Response bias can distort the actual findings of a study, necessitating careful design and execution of data collection methodologies.
By attentively addressing response bias, agricultural researchers can gather more accurate insights, further enabling informed decision-making in the sector. Understanding these challenges is foundational to improving the methodologies utilized in agricultural research.
Data Analysis Techniques
Data analysis techniques are a critical component of primary data collection in agriculture and horticulture research. Understanding how to analyze data effectively can greatly influence the outcomes of a study. Data analysis allows researchers to draw meaningful conclusions from raw data, guiding decisions that impact farming practices, crop yields, and sustainability initiatives. The correct analysis methods can unveil patterns and relationships that are not evident at first glance. Therefore, it is vital to select analysis techniques that align with the nature of the data collected and the objectives of the research.
Qualitative Data Analysis
Qualitative data analysis involves interpreting non-numerical data, such as interviews, focus groups, or open-ended survey responses. This type of analysis seeks to understand the underlying themes and patterns within the data. Methods like thematic analysis or content analysis are commonly used in this context.
Key steps in qualitative data analysis include:
- Transcription: Converting recorded interviews or discussions into written format.
- Coding: Identifying significant pieces of data and classifying them into themes or categories.
- Analyzing: Reviewing the codes to unearth broader insights and patterns.
Qualitative analysis can provide rich, in-depth views of participants' experiences and opinions. For example, a study examining farmer perceptions of sustainable practices may yield insights that are not quantifiable but are crucial for understanding motivations and barriers in agricultural innovation.
Quantitative Data Analysis
Quantitative data analysis focuses on numerical data, offering a powerful approach to evaluate and interpret findings. This method often employs statistical tools and software to manage complex datasets. Common techniques include descriptive statistics, inferential statistics, and regression analysis.
Some key processes involved in quantitative data analysis are:
- Data Entry: Inputting data into statistical software like SPSS or R for analysis.
- Descriptive Statistics: Summarizing data through means, medians, and standard deviations to understand distribution.
- Inferential Statistics: Drawing conclusions about a larger population based on sample data, often involving hypothesis testing.
The strength of quantitative analysis lies in its ability to produce measurable results that can support or refute a hypothesis. For example, analyzing crop yield data across multiple farms can reveal performance trends and help identify the factors contributing to high yields.
Case Studies in Primary Data Collection
Understanding the significance of case studies in primary data collection is crucial for research in agriculture and horticulture. Case studies offer a method to explore complex issues in real-life contexts. This approach helps researchers evaluate how various methods can be applied in the field. They provide insights into practical applications, demonstrate tangible outcomes, and highlight potential pitfalls. Utilizing case studies can enhance the effectiveness of future data collection strategies, making them invaluable for researchers and practitioners.
"Case studies serve as a bridge between theory and practice, facilitating a deeper understanding of agricultural challenges."
Successful Field Studies
Successful field studies exemplify effective primary data collection. They provide a framework for implementing data collection methods in agricultural scenarios. For instance, a study on organic farming practices can demonstrate how surveys and interviews yield comprehensive insights. Researchers often face varying conditions in agricultural contexts, such as soil types and climate. By analyzing successful cases, one can identify key factors that lead to valid data collection. These factors may include well-defined research objectives, appropriate sampling techniques, and thorough planning.
Successful case studies often incorporate a mixed-method approach. For example, combining qualitative insights from interviews with quantitative findings from experiments can provide a fuller perspective. The successful integration of multiple data sources can enhance the credibility of the findings, making the research more robust.
Lessons Learned from Failures
Not all case studies are successful. Examining the lessons learned from failures can provide valuable insights into what can go wrong in primary data collection. Failures might stem from poor planning, inadequate sampling methods, or the inability to address participant biases. For instance, a study might have faced challenges due to a limited sample size or a lack of diversity among participants, leading to skewed results.
Analyzing failed case studies highlights the importance of continual evaluation. Researchers must be open to revising their approaches based on previous experiences. This often includes improving data collection techniques, refining research questions, and ensuring ethical standards in participant engagement.
Failures also emphasize the necessity of training for those conducting data collection. Individuals without proper training may struggle to effectively collect and analyze data, leading to unreliable results. This highlights that the personnel involved in data collection are as crucial as the methods used.
Future Trends in Data Collection
The field of agriculture and horticulture is evolving rapidly, shaped by advancements in technology and changing environmental considerations. This section examines future trends in data collection, emphasizing the relevance and potential impact on farming practices. Improved data collection methods can significantly enhance the precision of research, thus fostering sustainable practices. As the agricultural sector faces challenges like climate change, population growth, and resource depletion, adopting innovative data collection approaches becomes essential to ensure effective responses.
Technological Innovations
Technological innovations are reshaping how data is collected in agriculture. Tools like satellite imagery, drones, and sensors are now utilized to gather data over vast areas with minimal human intervention. These advancements bring notable advantages:
- Precision Agriculture: Technologies allow farmers to measure soil moisture, crop health, and nutrient levels accurately. This precision minimizes waste and maximizes productivity.
- Data Analytics: Big Data analytics can process vast amounts of information quickly. It helps in identifying patterns and predicting outcomes, which informs better decision-making.
- Remote Monitoring: With the internet of Things (IoT), farmers can monitor conditions in real-time. This capability leads to timely interventions and improved yield.
Moreover, using mobile applications for data collection has simplified the process. These developments not only boost efficiency but also reduce labor costs.
Sustainability Focus
Sustainability in agriculture is becoming increasingly critical, and data collection methods play a significant role in this transition. Collecting data with a sustainability focus involves several aspects:
- Resource Optimization: Efficient data collection allows farmers to use resources like water and fertilizers more judiciously, thereby reducing environmental impact.
- Biodiversity Monitoring: Data helps track various species and ecosystems within agricultural settings, crucial for preserving biodiversity. Farmers can make informed choices about crop rotations and pest management.
- Climate Resilience: Understanding data related to weather patterns and soil health can aid farmers in adapting their practices to changing climates, ensuring long-term viability.
In summary, modernizing data collection through technological innovations and a focus on sustainability enhances both agriculture's productivity and ecological responsibility. This approach not only addresses present challenges but also prepares the sector for future demands.
Closure
Summary of Key Points
In summary, this article has discussed various primary data collection methods including surveys, interviews, observational methods, and experimental designs. Each of these methods has its unique strengths and weaknesses.
- Surveys allow for extensive data gathering from a broad audience, enabling researchers to filter responses efficiently.
- Interviews provide deeper insights but may be time-consuming and dependent on the interviewer’s skills.
- Observational methods capture real-time data, presenting an unfiltered look at agricultural environments.
- Experimental methods can be rigorous and controlled, fostering a clear understanding of cause-and-effect relationships.
Implementing these methods appropriately contributes to enhancing data validity and fostering sustainability in agricultural practices.
Implications for Practice
The implications of adopting effective primary data collection methods are extensive. Farmers and researchers gain not only better insights but also the capacity to tailor practices that contribute to sustainability and efficiency. For instance, using surveys can help farmers understand consumer preferences, leading to better product alignment in the market. Moreover, insights from experimental methods may pave the way for adopting innovative technologies and practices.